Prediction of an outcome using NETwork Clusters (NET-C) |
| |
Affiliation: | 1. Institute for Quantitative Biomedical Sciences, Dartmouth College, Hanover, NH;2. Department of Biomedical Data Science, Geisel School of Medicine, Lebanon, NH;3. Department of Biological Sciences, Dartmouth College, Hanover, NH;4. Department of Epidemiology, Geisel School of Medicine, Lebanon, NH;1. Tuberculosis and Lung Diseases Research Center, Tabriz University of Medical Sciences, P.O. Box: 53714161, Tabriz, Iran;2. Razi Vaccine and Serum Research Institute, Agricultural Research, Education and Extension Organization, Karaj, Iran;3. Department of Computer Engineering, Faculty of Electrical and Computer Engineering, University of Tabriz, Tabriz, Iran;4. Department of Molecular and Cell Biology, Faculty of Basic Sciences, University of Tehran, Tehran, Iran;5. Department of Life Science Engineering, Faculty of New Sciences and Technologies, University of Tehran, Tehran, Iran;6. Department of Interdisciplinary Life Sciences, University of Veterinary Medicine Vienna, Vienna, Austria;7. Departments of Environmental Medicine and Microbiology and Immunology, University of Rochester Medical Center, Rochester, NY, USA;1. Molecular Bio-Computation and Drug Design Laboratory, School of Health Sciences, University of KwaZulu-Natal, Westville Campus, Durban, 4001, South Africa;2. Department of Veterinary Parasitology and Entomology, Faculty of Veterinary Medicine, University of Ibadan, Nigeria;1. Department of Genetic Engineering & Biotechnology, Shahjalal University of Science & Technology, Sylhet, 3114, Bangladesh;2. Department of Medical Genetics, Faculty of Medicine and Dentistry, University of Alberta, 8440 112 St. NW, Edmonton, AB, T6G 2R7, Canada;1. Donald Danforth Plant Science Center, St. Louis, MO 63132, United States;2. Department of Biological Sciences, Dartmouth College, Hanover, NH 03755, United States;1. Department of Pathogenic Biology, School of Basic Medicine, Qingdao University, 308 Ningxia Road, Qingdao, 266021, China;2. Qingdao Shinan District Center for Disease Control and Prevention, 90 Xuzhou Road, Qingdao, 266021, China;3. Department of Blood Transfusion, The Affiliated Hospital of Qingdao University, 19 Jiangsu Road, Qingdao, 266021, China;1. Thayer School of Engineering, Dartmouth College, Hanover, NH 03755, USA;2. Department of Biology and Health Science, New England College, Henniker, NH 03242, USA;3. Department of Biological Sciences, Dartmouth College, Hanover, NH 03755, USA |
| |
Abstract: | 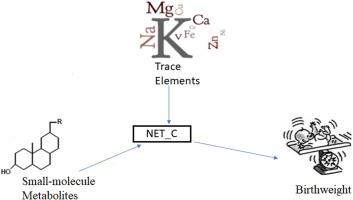 Birth weight is a key consequence of environmental exposures and metabolic alterations and can influence lifelong health. While a number of methods have been used to examine associations of trace element (including essential nutrients and toxic metals) concentrations or metabolite concentrations with a health outcome, birth weight, studies evaluating how the coexistence of these factors impacts birth weight are extremely limited. Here, we present a novel algorithm NETwork Clusters (NET-C), to improve the prediction of outcome by considering the interactions of features in the network and then apply this method to predict birth weight by jointly modelling trace element and cord blood metabolite data. Specifically, by using trace element and/or metabolite subnetworks as groups, we apply group lasso to estimate birth weight. We conducted statistical simulation studies to examine how both sample size and correlations between grouped features and the outcome affect prediction performance. We showed that in terms of prediction error, our proposed method outperformed other methods such as (a) group lasso with groups defined by hierarchical clustering, (b) random forest regression and (c) neural networks. We applied our method to data ascertained as part of the New Hampshire Birth Cohort Study on trace elements, metabolites and birth outcomes, adjusting for other covariates such as maternal body mass index (BMI) and enrollment age. Our proposed method can be applied to a variety of similarly structured high-dimensional datasets to predict health outcomes. |
| |
Keywords: | Outcome prediction Gaussian graphical model Lasso Dimensionality reduction Trace element exposures Metabolic network |
本文献已被 ScienceDirect 等数据库收录! |
|